Research
Artificial Intelligence in Neurological Disorders
Artificial Intelligence (AI) is profoundly transforming medical science, serving as a pivotal tool for predictive diagnosis and outcomes, particularly in the field of neurosurgical disorders. Our research employs advanced AI models, including machine learning, ensemble methods, and deep learning, to not only diagnose complex neurological conditions but also predict optimal treatment options or surgical outcomes and identify key biomarkers essential for these predictions. We focus on conditions such as Chiari malformation, skeletal dysplasia, syringomyelia, spina bifida, hydrocephalus, and brain tumors. By harnessing the capabilities of AI, we aim to advance personalized patient care, facilitate informed decision-making, and enhance outcomes in neurosurgery.

Comprehensive Morphometric Analysis to Identify Key Neuroimaging Biomarkers for the Diagnosis of Adult Hydrocephalus Using Artificial Intelligence
Hydrocephalus involves abnormal cerebrospinal fluid accumulation in brain ventricles. Early and accurate diagnosis is crucial for timely intervention and preventing progressive neurological deterioration. The aim of this study was to identify key neuroimaging biomarkers for the diagnosis of hydrocephalus using artificial intelligence to develop practical and accurate diagnostic tools for neurosurgeons.
Published Basic Science Research
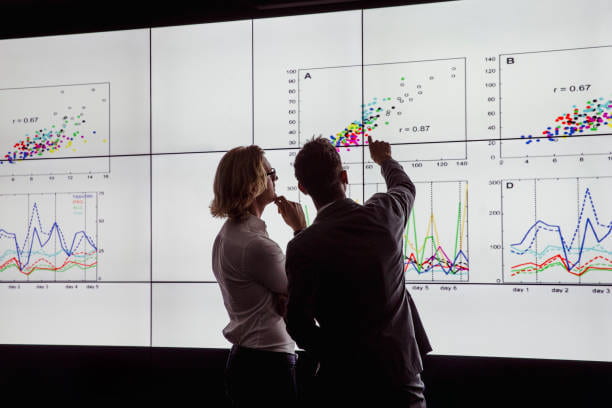
How We Predict and Improve Shunt Outcomes?
Cerebrospinal fluid shunt failure in patients with hydrocephalus is a complex process. This study aims to use the MHFP database to evaluate various factors that regulate shunt outcomes.
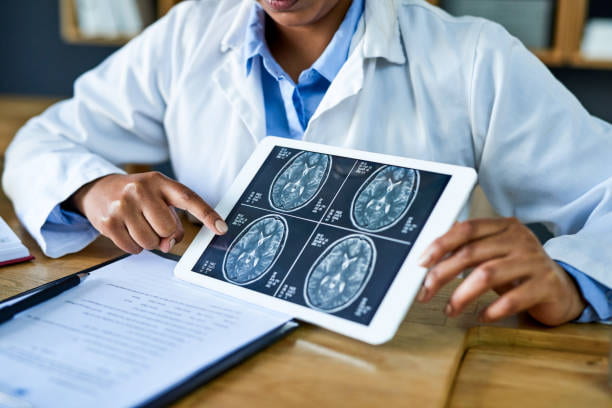
Hydrocephalus: Can Intracranial Compliance Predict Clinical Oscillations?
The impact of this study can gain insight into the quantitative evaluation of material property and relaxation behavior of brain tissue and their relationships with diagnosis, prognosis, and management of hydrocephalus patients.

Chiari
Chiari malformation type 1 (CM1) is a congenital anomaly of the cerebellum — the part of brain located at the base of the skull and brain stem.
Craniocervical junction morphology has been associated with Chiari malformation type I (CMI) symptom
severity; however, little is known about its deterministic effect on surgical outcomes in patients across age and sex differences. The goal of the present study was to assess the effects of age and sex on surgical outcomes in CMI.
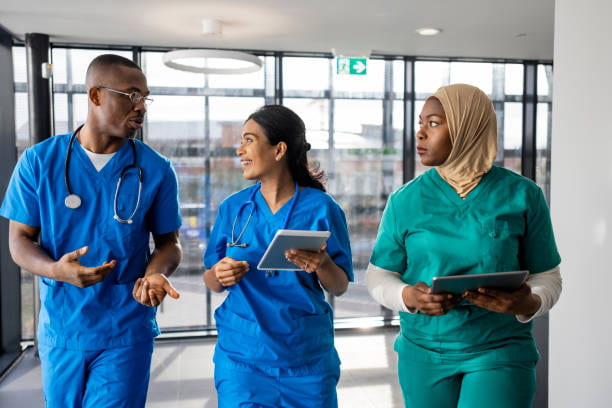
Special Considerations in Pediatric Endoscopic Skull Base Surgery
Originally pioneered in adults, endoscopic endonasal approaches for skull base pathology are being increasingly applied as a minimally invasive alternative for young children.
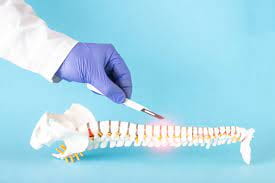
Questions to Solve When Blood, Brain & Cerebrospinal Fluid Interact
This study tries to understand the interaction of cerebral blood and cerebrospinal fluid with brain tissue to alleviate the pathophysiological ambiguities in cerebrospinal fluid dyscrasia.
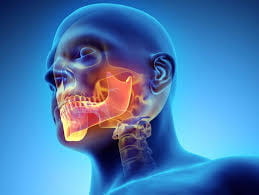
Skull Base Drilling: How Coolants Can Reduce Thermal & Physical Damage?
This project studies novel gas coolants to decrease the risk of thermal death in cells of the skull, blood coagulation, adjacent nerves injury, physical bone damage and micro-fractures during skull base drilling.

A review of intracranial aneurysm imaging modalities, from CT to state-of-the-art MR
This study review provides an organized, up-to-date summary on the array of available modalities/sequences for IA imaging to help build protocols focused on IA characterization.
Meet the MHFP Clinical Research Consortium
The MHFP Consortium consists of five pediatric neurosurgeons from various institutions around the country that focus research on Central Nervous System Anomalies such as achondroplasia, tethered cord, congenital brain tumors, skeletal dysplasias and more! Our current collaborating sites are Texas Children’s Hospital (Baylor College of Medicine), Boston Children’s Hospital (Harvard University), Cedars Sinai Medical Center, OHSU Doernbecher Children’s Hospital (OHSU), St. Louis Children’s Hospital (Washington University) and UChicago Medicine (University of Chicago). Each consortium site focuses on research in addition to contributing data to the MHFP Database to build a CNS Anomaly Database that contains nationwide data that is accessible for research!
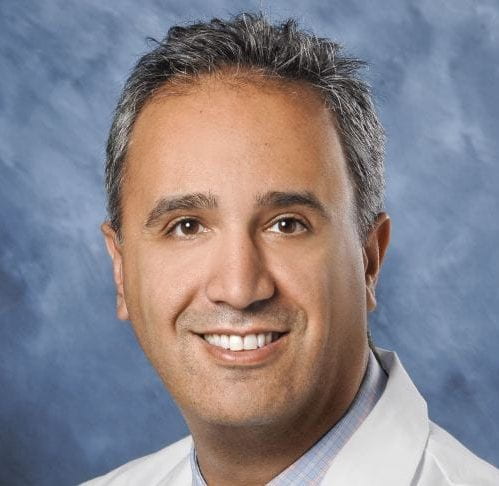
Moise Danielpour, MD
Neurosurgeon, Cedar-Sinai Medical Center, Los Angeles, CA
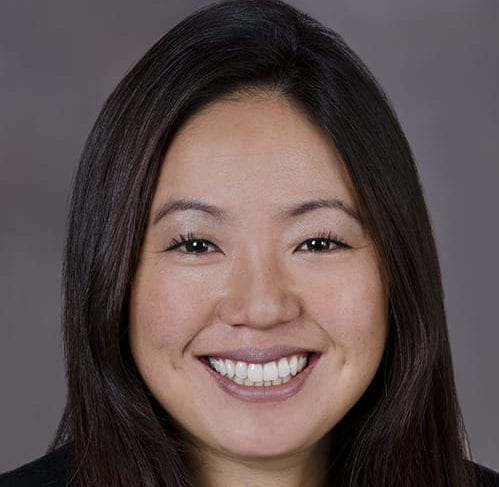
Christina Sayama, MD, MPH
Neurosurgeon, Oregon Health & Science University, Portland, OR

Eric Thompson, MD
Neurosurgeon, Washington University St. Louis, MO

Lissa Baird, MD
Neurosurgeon, Boston Children's Hospital, Boston, MA
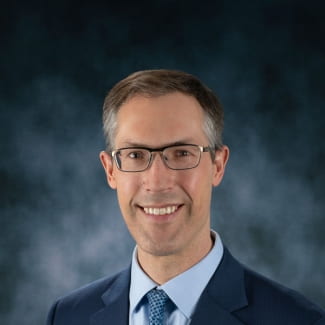
David Bauer, MD, MPH
Neurosurgeon, Baylor College of Medicine, Houston, TX

Bakhtiar Yamini, MD
Interim Chair, Dept. of Neurological Surgery, UChicago, Chicago, IL
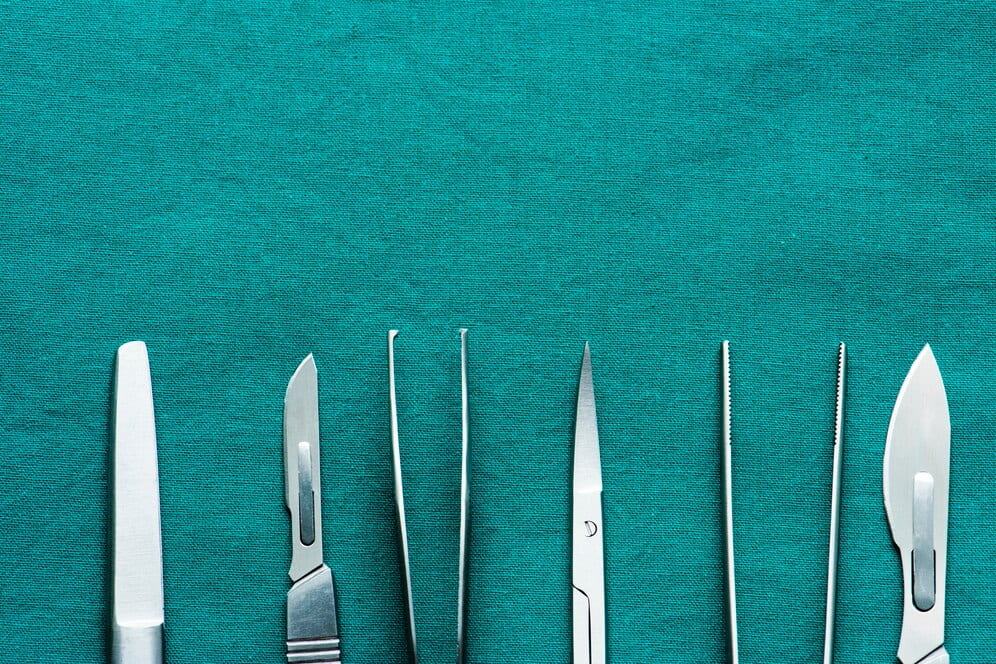
Skeletal Dysplasias
Skeletal dysplasias, or osteochondroplasias, encompass hundreds of disorders which affect the growth, development, and maturity of bone and cartilaginous structures. The most common of these is achondroplasia which occurs in around 1/25,000 births.

Achondroplasia
An autosomal dominant disorder that is the most frequent form of short-limb dwarfism. Affected individuals exhibit short stature caused by rhizomelic shortening of the limbs, characteristic facies with frontal bossing and mid-face hypoplasia, exaggerated lumbar lordosis, limitation of elbow extension, GENU VARUM, and trident hand.

Chiari
The traditional management of pregnant patients with Chiari I malformation (CM-I) heavily favors cesarean section and general anesthesia to limit the perceived risk of maternal neurological complications attributed to vaginal delivery and neuraxial anesthesia. The aim of this study was to compare reported neurological symptoms and radiographic presentations before and after childbirth performed by patients with CM-I, with and without associated syrinx.

MEET DR. SEIF GHOLAMPOUR
Research Professional, MHFP (Basic Science Research)
Dr. Seif Gholampour is a professional researcher for the MHFP at the Univ. of Chicago. He has over 14 years of research experience in Biomedical Modeling of CNS Disorders, Machine and Deep Learning in CSF Anomalies, Neuroinformatics, Image Processing in Neuroimaging, Image-based AI, Cerebral Blood Hemodynamics, CSF Hydrodynamics, and Image-based Computational Fluid Dynamics and Fluid-Structure Interaction Simulations in Neuroscience. His current projects focus on the diagnosis and evaluation of treatment options in CNS disorders using AI.
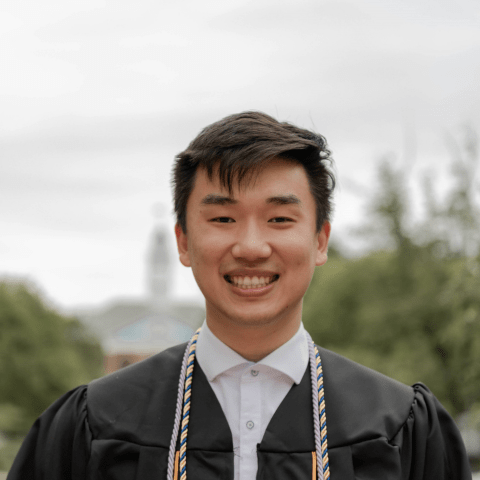
MEET JESSE HUANG
Senior Clinical Research Analyst, MHFP (Clinical Research)
As Senior Research Analyst at MHFP, Jesse leads clinical studies across all five of our national contributing hospitals. His work centers on designing and maintaining patient databases, mentoring study teams, and overseeing scientific writing. Jesse’s aptitude for integrating life sciences with data-driven insights, combined with his coding expertise, has been instrumental in driving MHFP’s burgeoning research productivity.
Committed to improving patients’ lives, Jesse also dedicates time to volunteering with organizations such as CommunityHealth and KEEN Chicago, supporting underserved communities throughout the city.
Prior to joining MHFP, Jesse earned dual bachelor’s degrees in Biophysics and Applied Mathematics & Statistics from the Johns Hopkins University. He has been published in a number of scientific publications and received invitations to present at several conferences.